August 13, 2016

Threat intelligence (TI) feeds, the way we know them today, are going to go away. In the future it’s all about fast and sometimes anonymous sharing of IOCs with trusted (and maybe untrusted) peers. TI feeds will probably stay around, but will be used for contextul information around our data.
For the past couple of years threat intelligence feeds (IOCs) emerged as a theme (hype) to help with threat detection. It almost seems like people got too tired of writing SIEM correlation rules and were hoping that IOCs would help them getting their job done much easier. There are many reasons that this approach isn’t working and won’t work going forward:
- Threat intelligence feeds are not complete. A number of people have been analyzing threat feeds and found that there is generally not much overlap in the different feeds (maybe 3-5%). What that also means is that each of the feed providers looks at a very different universe. And if that is true, what are we not seeing? If we saw more, we’d have much more overlap in the feeds.
- TI feeds or indicator matching is not the same as authoring correlation rules. Correlation rules can have complex temporal and spatial logic. Use it!
- IOCs are generally only valid for seconds or minutes. A machine gets infected, acts maliciously, and after a few minutes, the attacker already abandons it, just to replace it with a new one. What’s the delay within your TI feed? From the vendor actually putting the indicator on the feed to you being able to match it against your data?
So, what’s the future of TI then? Here are my three predictions:
- We need to enable real-time sharing of indicators. If I see something bad, I need to be able to share it with my peers immediately. Yes, this is challenging. But start small with a trusted group of peers. In some circumstances you might be okay sharing entire PCAPs. In others, just share the source of the attacks. Use an exchange mechanism that can help keeping the data source anonymous. It’s about speed and freshness of the IOCs.
- We can already see signs that threat intel providers are adding ‘contextual‘ information into their feeds. Things like what IP addresses are known TOR exit nodes, for example. Or what machines are part of a CDN. Useful? Probably some. What it really comes back to is asset context for your analytics and correlations. Knowing what a machine is can be incredibly useful. If you know that machine X is a DNS server and that only, why does it suddenly offer up Web traffic? So TI feeds will move more and more into contextual land.
- The ISACs will keep operating and help enable the exchange of TI among industry peers. We need to expand that concept outside of the ISACs as well. You will see more and more of the security providers that have a large enough customer base look into cross-customer intelligence. *cough* *cough*.
Keeping all of this in mind and run a serious trial before you buy any TI feed.
For a different look at Threat Intelligence – from a company internal perspective, read my blog on Internal Threat Intelligence.
Final thought / question: Has anyone leveraged threat intelligence feeds to come up with a weather report of the Internet and then adjusted their internal defense mechanisms accordingly?
What’s your experience, your thoughts around all this?
February 24, 2016
Okay, this post is going to be a bit strange. It’s a quick brain dump from a technical perspective, what it would take to build the perfect security monitoring environment.
We’d need data and contextual information to start with:
Any data:
- infrastructure / network logs (flows, dns, dhcp, proxy, routing, IPS, DLP, …)
- host logs (file access, process launch, socket activity, etc.)
- HIPS, anti virus, file integrity
- application logs (Web, SAP, HR, …)
- metrics
- configuration changes (host, network equipment, physical access, applications)
- indicators of compromise (threat feeds)
- physical access logs
- cloud instrumentation data
- change tickets
- incident information
Any context:
- asset information and classification
- identity context (roles, etc.)
- information classification and location (tracking movement?)
- HR / presonell information
- vulnerability scans
- configuration information for each machine, network device, and application
With all this information, what are the different jobs / tasks / themes that need to be covered from a data consumption perspective?
- situational awareness / dashboards
- alert triage
- forensic investigations
- metric generation from raw logs / mapping to some kind of risk
- incident management / sharing information / collaboration
- hunting
- running models on the data (data science)
-
- anomaly detection
- behavioral analysis
- scoring
- reports (PDF, HTML)
- real-time matching against high volume threat feeds
- remediating security problems
- continuous compliance
- controls verification / audit
What would the right data store look like? What would its capabilities be?
- storing any kind of data
- kind of schema less but with schema on demand
- storing event data (time-stamped data, logs)
- storing metrics
- fast random access of small amounts of data, aka search
- analytical questions
- looking for ‘patterns’ in the data – maybe something like a computer science grammar that defines patterns
- building dynamic context from the data (e.g., who was on what machine at what time)
- anonymization
Looks like there would probably be different data stores: We’d need an index, probably a graph store for complex queries, a columnar store for the analytical questions, pre-aggregated data to answer some of the common queries, and the raw logs as well. Oh boy 😉
I am sure I am missing a bunch of things here. Care to comment?
February 22, 2016
A week ago I was presenting at the Kaspersky Security Analyst Summit. My presentation was titled: “Creating Your Own Threat Intel Through Hunting & Visualization“.
Here are a couple of impressions from the conference:
Here I am showing some slides where I motivate why visualization is crucial for security analysts.
And a zoom in of the reason for why visualization is important. Note that emerging blue pattern towards the right of the scatter plot on the left. On the right you can see how context was used to augment the visualization to help identify outliers or interesting areas:
On the left here you see how visualization is used to find patterns and translate what you learn into algorithmic detections. On the right, I am showing a way to set thresholds on periodic data.
February 9, 2016
Hunting has been a fairly central topic on this blog. I have written about different aspects of hunting here and here.
I just gave a presentation at the Kaspersky Security Analytics Summit where I talked about the concept of internal threat intelligence and showed a number of visualizations to emphasize the concept of interactive discovery to find behavior that really matters in your network.
February 2, 2016
Recently I have been getting a number of questions about dashboards. I have written about them before (here and here). Given all the questions and a couple of recent consulting gigs where I built dashboards for different companies and purposes, I wanna share some simple learnings.
There are many different types of visualizations you can put on a dashboard: line charts, pie charts (or not), bar charts, bullet graphs, spark lines, maybe even treemaps. Keep in mind though that often a table is the best way to communicate your data.
Aside from tables, I have been using Netflix’s Hystrix visualizations more and more.
I really like them for dashboards for the following reasons:
- Adopt well to different scales (just like sparklines).
- Have multiple cognitive layers:
- If you have a larger dashboard, you can look at the different layers individually: The dots communicate the overall state, the solid line the progression over time, and the details can be shown as text for the curious mind.
- Mimic a traffic light methodology but without wasting space. Simple red, yellow, green circles are used to communicate the overall status.
- Include a time-series aspect to not only communicate the current state, but the development over time. Great to spot trends and get historic comparison.
- We can show the same metric from a previous time period (the dotted line). Again, great for comparison and baselining.
- Individual data points can be added as text.
- Don’t need absolute scales to make sense. The size of the circles can be used to communicate proportions.
- We can easily show causality (the heartbleed line), just like Edward Tufte has taught us.
Here is a quick simplified mockup of a dashboard that uses multiple charts of this type. As a bonus I included a bullet graph that can be used, for example, for benchmarking.
There is obviously more that goes into the design of a dashboard and the one shown here is really just a super raw starting point. The main challenge is always to determine the right security metrics that bubble up into the graphs. Turns out that’s generally really hard.
If this is the kind of thing you are interested in? Visualization for security, big data, security analytics, join me at BlackHat US in August, where I will be teaching the Visual Analytics Workshop. Sign up today!
October 16, 2015
Hunting is about learning about, and understand your environment. It is used to build a ‘model’ of your network and applications that you then leverage to configure and tune your detection mechanisms.
I have been preaching about the need to explore our security data for the past 9 or 10 years. I just went back in my slides and I even posted a process diagram about how to integrate exploratory analysis into the threat detection life cycle (sorry for the horrible graph, but back then I had to use PowerPoint 🙂 ):
Back then, people liked the visualizations they saw in my presentations, but nobody took the process seriously. They felt that the visualizations were pretty, but not that useful. Fast forward 7 or 8 years: Everyone is talking about hunting and how they need better tools an methods to explore their data. Suddenly those visualizations from back then are not just pretty, but people start seeing how they are actually really useful.
The core problem with threat detection is a broken process. Back in the glory days of SIEM, experts (or shall we say engineers) sat in a lab cooking up correlation rules and event prioritization formulas to help implement an event funnel:
This is so incredibly broken. [What do I know about this? I ran the team that did this for a big SIEM.] Not every environment is the same. There is no one formula that will help prioritize events. The correlation rules only scratch the surface, etc. etc. The fallout from this is that dozens of companies are frustrated with their SIEMs. They are looking for alternatives; throw them out and build their own. [Please don’t be one of them!]
Then threat intelligence came about. Instead of relying on signatures that identify common threats, let’s look for adversaries as well. Has that worked? Nope. There is a bunch of research indicating that the ‘external’ threat intelligence we have is not good.
What should we do? Well, we have to get away from thinking that a product will solve our problems. We have to roll-up our own sleeves and start creating our own ‘internal’ threat intelligence – through hunting.
The Hunting Process
Another word for hunting is exploring or learning. We need to understand our infrastructure, our applications to then find when there are things happening that are out of the norm.
Let’s look at the diagram above. What you see is a very simple data pipeline that we find everywhere. We ingest any kind of data. We then apply some kind of intelligence to the data to flag relevant events. In the SIEM case, the intelligence are mostly the correlation rules and the prioritization formula. [We have discussed this: pure voodoo.] Let’s have a look at the other pieces in the diagram:
- Context: This is any additional information we can gather about our objects (machines, users, and applications). This information is crucial for good intelligence. The more surprising it is that the SIEMs haven’t put much attention to features around this.
- IOCs: This is any kind of external threat intelligence. (see discussion above) We can use the IOCs to flag potentially bad activity.
- Interactive Visualization: This is really the heart of the hunting process. This is the interface that a hunter uses to explore and learn about the data. Flexibility, speed, and an amazing user experience are key.
- Internal TI: This is the internal threat intelligence that I mentioned above. This is basically anything we learn about our environment from the hunting process. The information is fed back into the overall system in the form of context and rules or patterns.
- Models: The other kind of intelligence or knowledge we gather from our hunting activities we can use to optimize and define new models for behavioral analysis, scoring, and finding anomalies. I know, this is kind of vague, but we could spend an entire blog post on just this. Ask if you wanna know more.
To summarize, the hunting process really helps us learn about our environment. It teaches us what is “normal” and how to find “anomalies”. More practically, it informs the rules we write, the patterns we try to find, the behavioral models we build. Without going through this process, we won’t be able to configure / define a system that has a fighting chance of finding advanced adversaries or attackers in our network. Non. Zero. Zilch.
Some more context and elaboration on some of the hunting concepts you can find in my recent darkreading blog post about Threat Intelligence.
October 12, 2015
VCs pay attention: There is an opportunity here, but it is going to be risky 😉 If you want to fund this, let me know.
In short: We need a company that builds and supports the data processing backend for all security products. Make it open source / free. And I don’t think this will be Cloudera. It’s too security specific. But they might have money to fund it? Tom?
I have had my frustrations with the security industry lately. Companies are not building products anymore, but features. Look at the security industry: You have a tool that does behavioral modeling for network traffic. You have a tool that does scoring of users based on information they extract from active directory. Yet another tool does the same for Linux systems and the next company does the same thing for the financial industry. Are you kidding me?
If you are a CISO right now, I don’t envy you. You have to a) figure out what type of products to even put in your environment and b) determine how to operate those products. We are back at where we were about 15 years ago. You need a dozen consoles to gain an understanding of your overall security environment. No cross-correlation, just an oportunity to justify the investment into a dozen screens on each analysts’ desk. Can we really not do better?
One of the fundamental problems that we have is that every single product re-builds the exact same data stack. Data ingestion with parsing, data storage, analytics, etc. It’s exactly the same stack in every single product. And guess what; using the different products, you have to feed all of them the exact same data. You end up collecting the same data multiple times.
We need someone – a company – to build the backend stack once and for all. It’s really clear at this point how to do that: Kafka -> Spark Streaming – Parquet and Cassandra – Spark SQL (maybe Impala). Throw some Esper in the mix, if you want to. Make the platform open so that everyone can plug in their own capabilities and we are done. Not rocket science. Addition: And it should be free / open source!
The hard part comes after. We need every (end-user) company out there to deploy this stack as their data pipeline and storage system (see Security Data Lake). Then, every product company needs to build their technology on top of this stack. That way, they don’t have to re-invent the wheel, companies don’t have to deploy dozens of products, and we can finally build decent product companies again that can focus on their core capabilities.
Now, who is going to fund the product company to build this? We don’t have time to go slow like Elastic in the case of ElasticSearch or RedHat for Linux. We need need this company now; a company that pulls together the right open source components, puts some glue between them, and offers services and maintenance.
Afterthought: Anyone feel like we are back in the year 2000? Isn’t this the exact same situation that the SIEMs were born out of? They promised to help with threat detection. Never delivered. Partly because of the technologies used (RDBMS). Partly due to the closeness of the architecture. Partly due to the fact that we thought we could come up with some formula that computes a priority for each event. Then look at priority 10 events and you are secure. Naive; or just inexperienced. (I am simplifying, but the correlation part is just an add-on to help find important events). If it weren’t for regulations and compliance use-cases, we wouldn’t even speak of SIEMs anymore. It’s time to rebuild this thing the right way. Let’s learn from our mistakes (and don’t get me started what all we have and are still doing wrong in our SIEMs [and those new “feature” products out there]).
June 21, 2015
I have been using MonetDB for a month now and have to say, I really like it. MonetDB is a columnar data store and it’s freaking fast.
However, when I started using it, I wasn’t on very good terms with my database. Here are the three things you have to know when you use MonetDB:
- Strings are quoted with single quotes. NOT double quotes! For example:
SELECT * FROM table where field like '%foo';
- Field names that are special terms need to be double quoted! There are many special terms, such as: “range”, “external”, “end”, … For example:
SELECT "end", other FROM table;
- The WHERE clause in a GROUP by statement is called HAVING (that’s just basic SQL knowledge, but good to remember): For example:
SELECT * FROM table GROUP BY field HAVING field>500;
- Querying an INET datatype needs to convert a STRING to an INET in the query as well:
SELECT * FROM table WHERE ip=inet '10.0.0.2';
or even cooler:
SELECT * FROM table WHERE ip<<inet '10.2.0.0/16';
- MonetDB has schemas. They are almost like different databases if you want. You can switch schema by using:
set schema foo;
- Inserting millions of records is fastest with the COPY INTO command:
cat file | mclient -d databse -s "COPY INTO table FROM STDIN using delimiters '\t' " -
- And here is how you create your own database and user:
sudo monetdb create _database_ -p _password_
sudo monetdb release _database_
mclient -u monetdb -d _database_
alter user set password '_password_' using old password 'monetdb';
create user "_username_" with password '_password_' name '_name_' schema "sys";
create schema "_your_schema_" authorization "_username_";
alter user _username_ set schema "_your_schema_";
I hope these things will help you deal with MonetDB a bit easier. The database is worth a try!!
June 7, 2015
Hunting in your security data is the process of using exploratory methods to discover insights and hopefully finding attacks that have previously been concealed. Visualization greatly simplifies and makes the exploratory process more efficient.
In my previous post, I talked about SIEM use-cases. I outlined how I go about defining detection use-cases. It’s not a linear process and it’s not something that is the same for every company. That’s also a reason why I didn’t give you a list of use-cases to implement in your SIEM. There are guidelines, but in the end, you need to build a use-case repository unique to your organization. In this post we are going to explore a bit more what that means and how a ‘hunting‘ capability can help you with that.
There are three main approaches to implementing a use-case in a SIEM:
- Rules: Some kind of deterministic set of conditions. For example: Find three consecutive failed logins between the same machines and using the same username.
- Simple statistics: Leveraging simple statistical properties, such as standard deviations, means, medians, or correlation measures to characterize attacks or otherwise interesting behavior. A simple example would be to look at the volume of traffic between machines and finding instances where the volume deviates from the norm by more than two standard deviations.
- Behavioral models: Often behavioral models are just slightly more complicated statistics. Scoring is often the bases of the models. The models often rely on classifications or regressions. On top of that you then define anomaly detectors that flag outliers. An example would be to look at the behavior of each user in your system. If their behavior changes, the model should flag that. Easier said than done.
I am not going into discussing how effective these above approaches are and what the issues are with them. Let’s just assume they are effective and easy to implement. [I can’t resist: On anomaly detection: Just answer me this: “What’s normal?” Oh, and don’t get lost in complicated data science. Experience shows that simple statistics mostly yield the best output.]
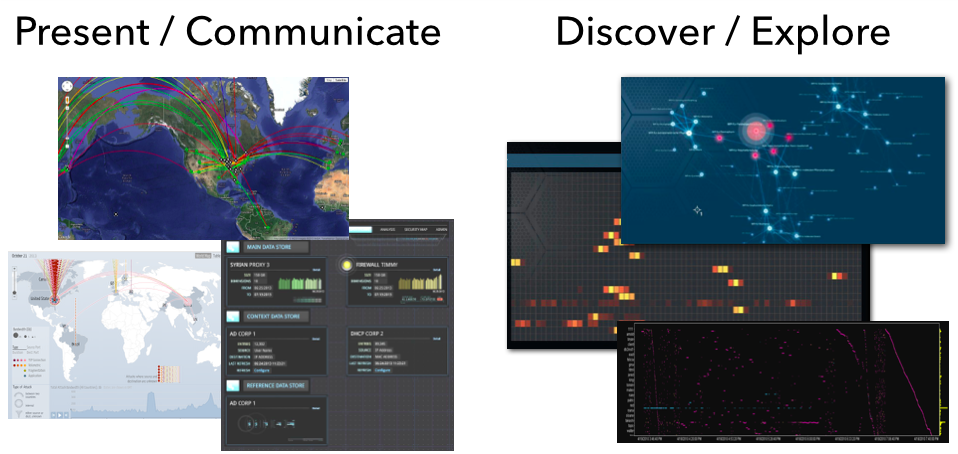
Let’s bring things back to visualization and see how that related to all of this. I like to split visualization into two areas:
- Communication: This is where you leverage visualization to communicate some property of your data. You already know what you want the viewer to focus on. This is often closely tied to metrics that help abstract the underlying data into something that can be put into a dashboard. Dashboards are meant to help the viewer gain an overview of what is happening; the overall state. Only in some very limited cases will you be able to use dashboards to actually discover some novel insight into your data. That’s not their main purpose.
- Exploration: What is my data about? This is literal exploration where one leverages visualization to dig into the data to quickly understand it. Generally we don’t know what we are going to find. We don’t know our data and want to understand it as quickly as possible. We want insights.
From a visualization standpoint the two are very different as well. Visually exploring data is done using more sophisticated visualizations, such as parallel coordinates, heatmaps, link graphs, etc. Sometimes a bar or a line chart might come in handy, but those are generally not “data-dense” enough. Following are a couple more points about exploratory visualizations:
- It important to understand that these approaches need very powerful backends or data stores to drive the visualizations. This is not Excel!
- Visualization is not enough. You also need a powerful way of translating your data into visualizations. Often this is simple aggregation, but in some cases, more sophisticated data mining comes in handy. Think clustering.
- The exploratory process is all about finding unknowns. If you already knew what you are looking for, visualization might help you identify those instances quicker and easier, but generally you would leverage visualization to find the unknown unknowns. Once you identified them, you can then go ahead and implement those with one of the traditional approaches: rules, statistics, or behaviors in order to automate finding them in the future.
- Some of the insights you will discover can’t be described in any of the above ways. The parameters are not clear or change ever so slightly. However, visually those outliers are quite apparent. In these cases, you should extend your analysis process to regularly have someone visualize the data to look for these instances.
- You can absolutely try to explore your data without visualization. In some instances that might work out well. But careful; statistical summaries of your data will never tell you the full story (see Anscombe’s Quartet – the four data series all have the same statistical summaries, but looking at the visuals, each of them tells a different story).
In cyber security (or information security), we have started calling the exploratory process “Hunting“. This closes the loop to our SIEM use-cases. Hunting is used to discover and define new detection use-cases. I see security teams leverage hunting capabilities to do exactly that. Extending their threat intelligence capabilities to find the more sophisticated attackers that other tools wouldn’t be able to identify. Or maybe only partly, but then in concert with other data sources, they are able to create a better, more insightful picture.
In the context of hunting, a client lately asked the following questions: How do you measure the efficiency of your hunting team? How do you justify a hunting team opposite an ROI? And how do you assess the efficiency of analyst A versus analyst B? I gave them the following three answers:
- When running any red-teaming approach, how quickly is your hunting team able to find the attacks? Are they quicker than your SOC team?
- Are your hunters better than your IDS? Or are they finding issues that your IDS already flagged? (substitute IDS with any of your other detection mechanisms)
- How many incidents are reported to you from outside the security group? Does your hunting team bring those numbers down? If your hunting team wasn’t in place, would that number be even higher?
- For each incident that is reported external to your security team, assess whether the hunt team should have found them. If not, figure out how to enable them to do that in the future.
Unfortunately, there are no great hunting tools out there. Especially when it comes to leveraging visualization to do so. You will find people using some of the BI tools to visualize traffic, build Hadoop-based backends to support the data needs, etc. But in the end; these approaches don’t scale and won’t give you satisfying visualization and in turn insights. But that’s what pixlcloud‘s mission is. Get in touch!
Put your hunting experience, stories, challenges, and insights into the comments! I wanna hear from you!
May 7, 2015
As it happens, I do a lot of consulting for companies that have some kind of log management or SIEM solution deployed. Unfortunately, or maybe not really for me, most companies have a hard time figuring out what to do with their expensive toys. [It is a completely different topic what I think about the security monitoring / SIEM space in general – it’s quite broken.] But here are some tips that I share with companies that are trying to get more out of their SIEMs:
- First and foremost, start with use-cases. Time and time again, I am on calls with companies and they are telling me that they have been onboarding data sources for the last 4 months. When I ask them what they are trying to do with them, it gets really quiet. Turns out that’s what they expected me to tell them. Well, that’s not how it works. You have to come up with the use-cases you want/need to implement yourself. I don’t know your specific environment, your security policy, or your threat profile. These are the factors that should drive your use-cases.
- Second, focus on your assets / machines. Identify your most valuable assets – the high business impact (HBI) machines and network segments. Even just identifying them can be quite challenging. I can guarantee you though; the time is well spent. After all, you need to know what you are protecting.
- Model a set of use-cases around your HBIs. Learn as much as you can about them: What software is running on them? What processes are running? What ports are open? And from a network point of view, what other machines are they communicating with? What internal machines have access to talk to them? Do they talk to the outside world? What machines? How may different ones? When? Use your imagination to come up with more use-cases. Monitor the machines for a week and start defining some policies / metrics that you can monitor. Keep adopting them over time.
- Based on your use-cases, determine what data you need. You will be surprised what you learn. Your IDS logs might suddenly loose a lot of importance. But your authentication logs and network flows might come in pretty handy. Note how we turned things around; instead of having the data dictate our use-cases, we have the use-cases dictate what data we collect.
- Next up, figure out how to actually implement your use-cases. Your SIEM is probably going to be the central point for most of the use-case implementations. However, it won’t be able to solve all of your use-cases. You might need some pretty specific tools to model user behavior, machine communications, etc. But also don’t give up too quickly. Your SIEM can do a lot; even initial machine profiling. Try to work with what you have.
Ideally you go through this process before you buy any products. To come up with a set of use-cases, involve your risk management people too. They can help you prioritize your efforts and probably have a number of use-cases they would like to see addressed as well. What I often do is organize a brainstorming session with many different stakeholders across different departments.
Here are some additional resources that might come in handy in your use-case development efforts:
- Popular SIEM Starter Use Cases – This is a short list of use-cases you can work with. You will need to determine how exactly to collect the data that Anton is talking about in this blog post and how to actually implement the use-case, but the list is a great starting point.
- AlienVault SIEM Use-Cases – Scroll down just a bit and you will see a list of SIEM use-cases. If you click on them, they will open up and show you some more details around how to implement them. Great list to get started.
- SANS Critical Security Controls – While this is not specifically a list of SIEM use-cases, I like to use this list as a guide to explore SIEM use-cases. Go through the controls and identify which ones you care about and how you could map them to your SIEM.
- NIST 800-53 – This is NIST’s control framework. Again, not directly a list of SIEM use-cases, but similar to the SANS list, a great place for inspiration, but also a nice framework to follow in order to make sure you cover the important use-cases. [When I was running the solution team at ArcSight, we implemented an entire solution (app) around the NIST framework.]
Interestingly enough, on most of my recent consulting calls and engagements around SIEM use-cases, I got asked about how to visualize the data in the SIEM to make it more tangible and actionable. Unfortunately, there is no tool out there that would let you do that out of the box. Not yet. Here are a couple of resources you can have a look at to get going though:
- secviz.org is the community portal for security visualization
- I sometimes use Gephi for network graph visualizations. The problem is that it is limited to only network graphs. Very quickly you will realize that it would be nice to have other, linked visualizations too. You are off in ‘do it yourself’ land.
- There is also DAVIX which is a Linux distro for security visualization with a ton of visualization tools readily installed.
- And – shameless plug – I teach log analysis and visualization workshops where we discuss more of these topics and tools.
Do you have SIEM use-cases that you find super useful? Add them in the comments!